What automation leaders need to know
AI agents are a new software paradigm enabled by LLMs. Agents can reason, collaborate, and act, similar to how humans work. Introduction to enterprise AI agents: What is an enterprise AI agent?
A decade after the rise of RPA, we are on the verge of a new shift in enterprise automation with intelligent AI agents powered by large language models (LLMs). Agents are not just an incrementally better way to automate but an entirely new breakthrough technology that requires new skills and tools to master. They expand the scope from tactical task-level automation to automating and augmenting complex knowledge work.
Moving from RPA to AI agents is a strategic initiative that requires executive-level sponsorship to succeed. At the same time, it is also an opportunity for automation leaders and CoEs to reimagine their role as strategic enablers inside the enterprise. In this article, we will explore some of the practical implications of jumping from RPA to enterprise AI agents.
The evolution of enterprise automation:
RPA automating | Tasks |
Automation as code automating | Processes |
Intelligent AI agents automating | Work |
Beyond RPA and intelligent automation
Enterprise automation is going through an evolution. It started out with Robotic Process Automation (RPA) and was popularized by companies like UiPath in the mid-2010s. RPA’s rise to fame was fueled by record-and-playback style UI automation and it helped to create the boom around low-code business apps.
Even though skeptics initially raised concerns about the fragility of RPA and the durability of bot automation, by now, every major company has established a center of excellence around automation and expanded to what’s commonly known as intelligent automation (IA).
IA is not a well-defined term, and often, it is a mix of techniques from RPA to API automation and document processing through OCR. It signals the move beyond point-and-click automation to process automation with tools that often mix coding with low-code and require specialist skills to deploy. However, the basic principles remain the same – RPA and IA are purely rule-based and suited for well-defined, highly structured processes.
AI agents, on the other hand, use a completely different agentic planning and execution workflow. They can natively understand unstructured data and unstructured processes through their flexible reasoning capabilities. This makes agents suitable for work that cannot be captured with simple rules but is described instead in natural language runbooks and working guides. It also makes agents more resilient than pre-programmed bots – agents can correct themselves if they hit and error or reach out to a human for feedback.
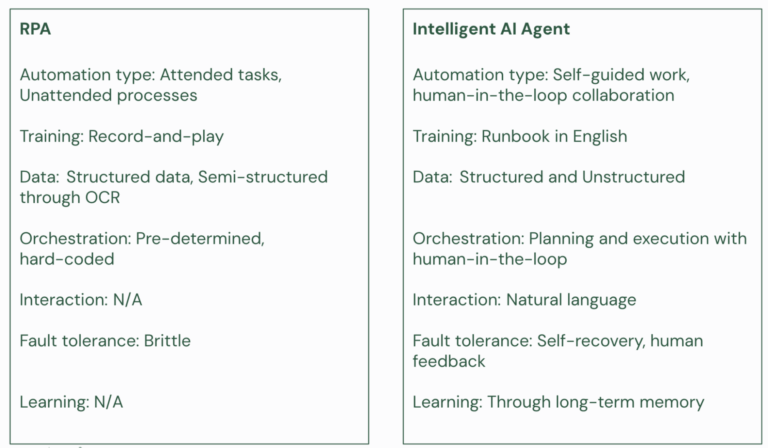
From tactical automation to strategic AI agents
Even though AI agents take a very different approach to enterprise automation, they do not mark the end of RPA. If your task requires entering a thousand records into a production ERP system daily or migrating a hundred thousand electronic health records to a new system, there’s no point in trying to fit an agent to solve a repetitive routine task. If all you have is a hammer, we know how that goes.
AI agents expand the scope of enterprise automation beyond what was previously possible. Since AI agents are a new category that is still taking shape, the easiest way to think about agent use-cases is to expand existing automation routines with agentic reasoning. Over time, AI agents will expand to strategic core business workflows that are at the heart of the enterprise.
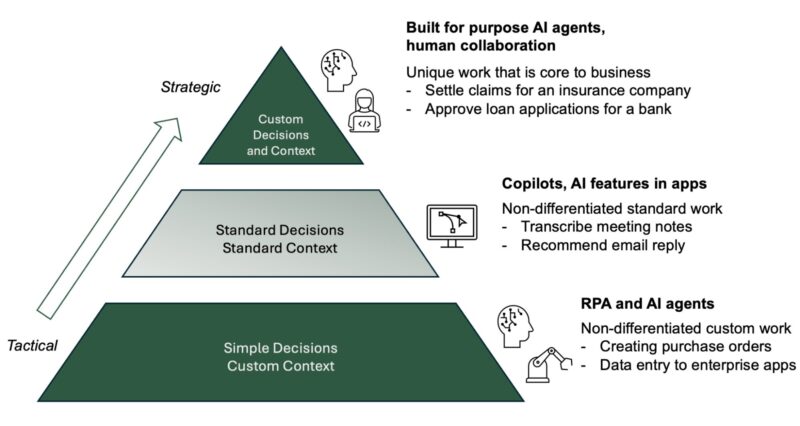
The illustration above shows three different categories of automation use-cases. At the bottom, there is a large amount of tactical work that does not require complex decision-making but runs on custom context. That is to say, this work operates on data, documents, and systems that are highly specific to the enterprise in question.
This is where RPA operates, and it’s a good place to start with the first AI agent projects. Look for opportunities in workflows that come before and after RPA routines and expand the scope of automation with agents. Use AI agents to grow the scope of automation beyond what’s possible with standard RPA.
Moving up the pyramid, we have work that involves standard decisions that operate on standard context. This represents work that is often captured by platforms and systems of record like ServiceNow or Salesforce. Here, you will be best served by buying AI and automation solutions from these vendors and relying on their ability to innovate on the data and processes they own. There’s a copilot for every app right now.
Finally, at the top of the pyramid we have the most strategic category of work that is central to how the enterprise does business and operates. This work involves complex decisions and it operates on custom context. Nobody will be able to come in from the outside to teach you how to do this work better because it is why the company exists. This is where built-for-purpose enterprise AI agents are going to have the biggest impact.
Agents can work alongside humans or replace some of our human tasks altogether and enable people to focus on more productive work. Since we, as an industry, are still early in our journey with intelligent AI agents, it will take time to get to this most valuable and strategic work, but right now is the right time to start building the skills and platforms that are needed to get there.
Three steps to get started on your journey from automation to AI
The current pace of change and innovation in AI can feel overwhelming, and we are often faced with the question of how we should even get started. Even before the first step, it is of course good to learn about AI agents and have a point of view on the technology. Here are two good resources to get started with that don’t require previous background in AI:
Now, to start on the enterprise AI agent journey, the first step is to:
- Establish AI governance and security guidelines
How do we control the use of AI, how do we allow our data to be exposed to AI applications, and how do we make sure AI doesn’t become a threat to security? This is the groundwork every enterprise has to do and set clear ground rules for AI experimentation. We have seen industry best practices emerge around AI governance, and this topic warrants its own writeup later.
- Define timeline and success criteria and align the organization for success
The second step is to set the right expectations, timeline, and strategic vision. “By the end of the year we have three AI agents identified and built, running in day-to-day operations. Our vision is to become an AI-enabled enterprise over the next two years and apply AI agents to our core business processes.” Starting with a clearly defined timeline and reasonable expectations allows the organization to learn and ramp up with agents and claim early victory. A strategic vision statement is needed to ensure we stay on course to make the right impact. Executive-level sponsorship is key to the success of the program.
- Identify use-cases, starting with tactical automation and working up to strategic
Look into the existing automation pipeline to identify the first AI agent use-cases, or look at what workstreams come before and after current automations. These are good places to start collecting ideas for agents and steer away from use-cases that fall into the middle layer of our use-case pyramid. Have a point of view about the most strategic use-cases for your company, but start small so that you can stay on the timeline and facilitate learning.
AI and agent technologies are rapidly developing with new breakthroughs and LLMs are coming out at a relentless pace. In order to stay relevant in this market, it is good to avoid locking into a technology or LLM vendor. Instead, look for vendors that leverage open-source technologies in their offerings since open-source is leading the innovation in AI agents.
The time to act is now
AI agents are new breakthrough technology, not an incremental step from traditional automation. Traditionally, the strategy around automation and AI has been to plan for the next big thing while navigating the present challenges. However, the pace at which generative AI evolves suggests a critical pivot in this approach. The future is not two years down the line; it is now.
As leaders in enterprise automation, it’s vital to shift focus from a distant horizon to the immediate implementation of AI technologies. Waiting to adapt means missing out on the competitive edge that AI offers today. There are many forward-looking companies already getting significant benefits from the AI programs they are building.
To stay up to date with enterprise AI agents, follow Sema4.ai and connect with us on LinkedIn.